Announcing:
Beyond the Science of Reading: Connecting Literacy Instruction to the Science of Learning
Wexler debunks common myths about how children learn to read, explores the connection between reading and writing skills, and offers practical solutions for bringing science-informed teaching to scale.
Wexler debunks common myths about how children learn to read, explores the connection between reading and writing skills, and offers practical solutions for bringing science-informed teaching to scale.
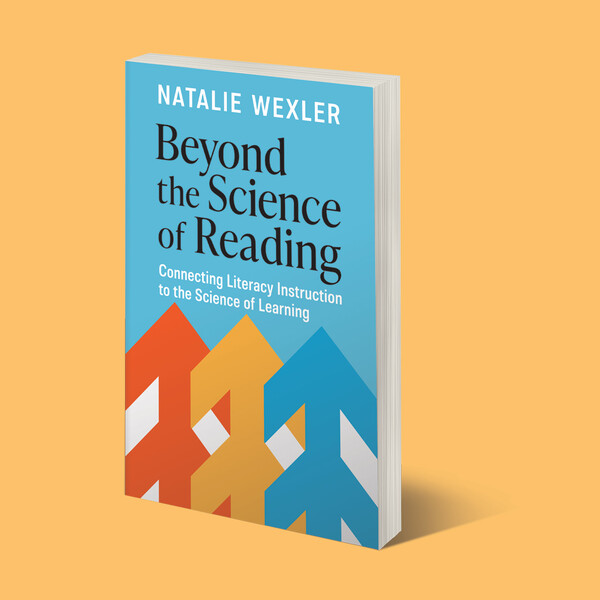
Premium members receive 9 new books a year. Join today
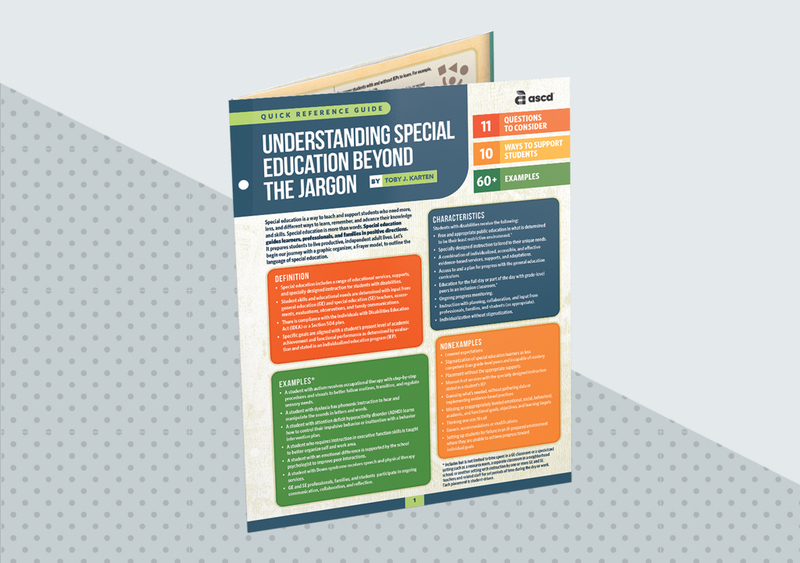